Deepfakes: A looming threat to financial markets? - Ololade Folashade
- Ololade Folashade
- Jun 12, 2024
- 7 min read
The transformative power of generative AI is undeniable. It has revolutionized industries like economics, healthcare, and technology by automating processes, predicting future trends, and ushering in a new era of intelligent machines. However, this very power presents a double-edged sword. Deepfakes, a subfield of generative AI capable of producing highly realistic synthetic media, pose a significant threat to financial markets. Deepfakes, a generative AI, leverage deep learning to create highly realistic synthetic media, like videos or audio recordings. This technology poses a significant threat to financial markets. As deepfakes become more sophisticated, concerns are rising about their potential to be used for malicious purposes, such as manipulating investors or disrupting market confidence. A recent case in Hong Kong, where a finance worker was tricked out of $25 million through a deepfake impersonation (Heather Chen, CNN World, 2024), highlights the real-world dangers of this technology. This research aims to explore the threats posed by deepfakes in financial markets, analyze their potential impact, and propose mitigation strategies for financial institutions.
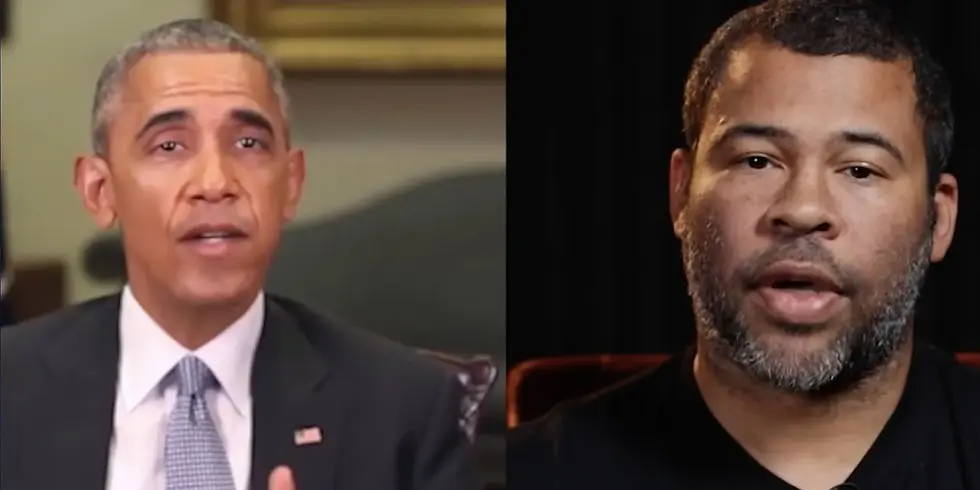
How Deepfakes Can Be Used for Market Manipulation:
The emergence of deepfakes, hyper-realistic synthetic media generated by artificial intelligence, poses a significant threat to financial markets. Deepfakes can erode investor confidence and disrupt market stability through various manipulation techniques. This research explores the potential avenues for deepfake misuse in financial markets, analyzing specific attack vectors and their potential impact on market participants.
Types of Deepfake Manipulation:
Impersonation: Deepfakes can be used to impersonate key financial figures like CEOs or company executives. Manipulated audio or video recordings could authorize fraudulent transactions or create misleading press releases, impacting investor sentiment and stock prices.
Business Email Compromise (BEC): Deepfakes can be leveraged to enhance existing BEC scams. Synthetic videos or emails seemingly originating from trusted sources within an organization could trick employees into authorizing unauthorized transfers or disclosing sensitive information.
Synthetic Financial Documents: Deepfakes can create fabricated financial statements, trading reports, or bank account records. These seemingly authentic documents could mislead investors or financial institutions, impacting lending decisions or investment strategies.
Scenario-Based Analysis: Deepfakes and Financial Threats
This section explores the potential application of deepfakes in perpetrating various financial crimes. Through scenario-based analysis, we aim to assess the threat landscape and identify critical areas for mitigation strategies.
Deepfakes in Payment Fraud. Deepfakes present a novel challenge to identity verification systems. Malicious actors could leverage this technology to manipulate facial recognition or voice authentication protocols, enabling them to impersonate individuals and authorize fraudulent transactions. The surge in deepfake-enabled fraud incidents, with a 1740% increase in North America between 2022 and 2023, underscores the urgency of addressing this vulnerability.
A recent case exemplified this risk. Criminals employed voice cloning technology to impersonate a German CEO, successfully tricking a British counterpart into authorizing a fraudulent wire transfer of $243,000. This incident highlights the potential for deepfakes to evolve beyond voice cloning and encompass realistic live video manipulation.
1
Stock Manipulation using Fabricated Events. Deepfakes can destabilize financial markets by creating fake news or manipulating existing footage to influence investor sentiment. Malicious actors could generate deepfakes that portray false narratives about a company, leading to a decline in its stock price. This creates a new vulnerability for companies to
1
https://www.statista.com/chart/31901/countries-per-region-with-biggest-increases-in-deepfake-specific -fraud-cases/
navigate, as fabricated statements from organizational leaders, even if eventually refuted, can inflict lasting reputational damage. Research suggests that a portion of viewers might still believe deepfakes despite warnings, further amplifying their potential impact. Additionally, deepfakes could erode consumer trust in companies, leading to long-term revenue and stock price declines, particularly for consumer-facing businesses. This threat extends to the realm of stock manipulation using bots, potentially amplifying the effect of deepfake-driven sentiment shifts.
Other forms of the misuse of deepfakes technologies include deepfakes and bank run, flash crashes via deepfake disinformation, pump and dump schemes, and short and distort Schemes.
Mitigating the Deepfake Threat in Financial Markets
The widespread adoption of deepfakes necessitates proactive measures from market regulators, financial institutions, and individual investors. This research will explore potential solutions to mitigate deepfake manipulation, such as enhanced authentication protocols, media literacy campaigns, and the development of deepfake detection technologies.
Addressing the Developed Market Assumption:
While some developed markets may possess robust technological defenses and financial buffers, dismissing deepfakes as a non-issue would be short-sighted. The ease of access and continuous improvement of deepfake technology warrants a proactive stance to safeguard financial integrity globally.
Proposed Solutions:
Advanced Video Analysis and Machine Learning. A critical component in the fight against deepfake-enabled financial fraud is the development of robust detection technologies. Investing in research and development of advanced video analysis and machine learning algorithms specifically designed to identify deepfakes is crucial. These technologies offer the potential for automated detection, enabling swift intervention and mitigation strategies. By leveraging image and video detection models, institutions can significantly enhance their preparedness to address the evolving threat of deepfakes.
Image Detection Models and Deep Neural Networks. Image detection models play a key role in deepfake identification. These models utilize deep neural networks, similar to those used in face recognition, to analyze facial features and identify inconsistencies indicative of manipulation. Several techniques hold promise in this domain, including:
Pair-wise Learning Models. To identify discrepancies, these models compare two images, a known genuine image and a suspected deepfake.Two-Stream Networks: This approach divides the analysis into two streams, focusing on spatial features and motion patterns, providing a more comprehensive assessment. Forensics Convolutional Neural Networks (FCNNs). FCNNs are specifically trained to detect subtle artifacts and inconsistencies often present in deepfakes, such as blurring or unnatural skin tones.
Video Detection Models and Biological Signals Analysis. Video detection models extend deepfake identification beyond static images. These models can analyze biological signals such as eye blinking patterns and heart rate fluctuations, which can be manipulated in deepfakes. Convolutional neural networks (CNNs) can be employed to analyze these subtle inconsistencies. Additionally, Siamese network-based architectures offer promise by simultaneously analyzing facial features and corresponding speech patterns, helping to identify inconsistencies that might arise during manipulation.
Continuous Improvement Through Technological Advancements. These detection models' ongoing development and refinement are crucial for staying ahead of deepfake creators. By leveraging advanced video analysis and machine learning, we can continuously improve our ability to identify and mitigate the risks posed by deepfakes in the financial sector.
Media Literacy and Education:
Equipping individuals with media literacy skills is crucial in the fight against deepfakes within the financial services sector. Educational campaigns can empower individuals to:
Identify Deepfakes in Financial Contexts. Educating the public on the red flags of deepfakes used in financial scams is essential. This includes recognizing unnatural movements, inconsistencies in lighting and skin tones in fabricated videos of officials, and glitches in video editing often present in deepfakes designed to manipulate financial news.
Verify Information Before Making Financial Decisions. Encouraging individuals to cross-reference information across multiple credible sources, particularly those issued by established financial institutions or regulatory bodies, before making investment decisions or engaging in financial transactions helps mitigate the impact of deepfake-based manipulation tactics.
Employee Training and Upstream Resilience. Financial institutions can further strengthen their defenses by:
Training Employees. Equipping employees with knowledge to identify deepfakes used in phishing attempts, social engineering scams targeting account information or wire transfers, and other fraudulent activities designed to exploit the financial system. Prevention-Detection-Response Framework. Implementing a structured approach emphasizing proactive measures like multi-factor authentication and user education on deepfakes. Additionally, it is crucial to have robust detection capabilities for early identification and a clear response protocol for mitigating their impact, such as account suspension or fraud investigation.
Collaboration with Marketing and Branding Partners. Raising awareness among marketing, PR, and branding partners can strengthen defenses against the spread of deepfake-based disinformation that could erode trust in the financial institution. This involves developing proactive strategies to address potential leaks of sensitive information and a unified approach with partners to combat misinformation that could impact financial markets. Empowering Customers with the Sift Reliance Framework
Banks can further empower their customers by promoting the Sift Reliance Framework.
Stop, Investigate, Find Trusted Coverage, and Trace Original Content. This framework can equip customers to identify potential data manipulation attempts, including those leveraging deepfakes, and take appropriate action to protect their financial information in case of a bank run or other threats.
Regulatory Policies:
There are currently no outright laws to ban the use or policies to ban the creation or use of deep fakes in the USA, so in the case of regulatory policies, frameworks are just evolving; however, some of the steps that can be taken by regulatory bodies to develop robust regulatory frameworks is essential. This could involve:
Disclosure Requirements. Requiring companies to disclose potential deepfake risks and outline reporting procedures for suspected deepfake manipulation attempts.Liability Frameworks. Establishing explicit legal liabilities for individuals or entities who intentionally create or utilize deepfakes for financial manipulation.
Collaboration. Fostering international cooperation among regulatory bodies to create a unified front against deepfakes in financial markets.
Conclusion:
In conclusion, deepfakes pose a significant threat to the stability and integrity of financial markets. Their ability to manipulate information and sow distrust can have a cascading effect, triggering bank runs, flash crashes fueled by disinformation, and eroding confidence in institutions. Deepfakes can also be used for targeted attacks, such as impersonating executives to authorize fraudulent transactions or manipulating stock prices through
fabricated events. The versatility of deepfakes makes them a growing concern for regulators and market participants alike. While encouraging advancements are being made in deepfake detection and mitigation, continuous vigilance and technological development remain paramount to safeguarding financial systems from this evolving threat.
Ethical Reference
Research content includes using AI tools like Wordtune Grammarly, AI to help improve grammatical errors or formatting. All content is based on original research from multiple sources.
Reference
Heather, C. (2024, February 4). Finance worker pays out $25M after video call with deep fake ‘CEO’ https://www.cnn.com/2024/02/04/asia/deepfake-cfo-scam-hong-kong-intl-hnk/index.html
Bryan, L. & Matt, T. (2023) Exploring Deepfakes (1st ed.). Packt Publishing Ltd.
Subham, T. (2023, November 24). Deepfake Stock Market Scamd: How AI is being used to trick investors https://www.businesstoday.in/technology/news/story/deepfake-stock-market-scams-how-ai- is-being-used-to-trick-investors-406972-2023-11-24?onetap=true
NSA, FBI, CISA: Conceptualizing Deepfake Threats to Organizations
https://carnegieendowment.org/2020/07/08/deepfakes-and-synthetic-media-in-financial-sy stem-assessing-threat-scenarios-pub-82237
Florian, Z. (2024, March 13) How Dangerous are Deepfakes and other AI Powered Fraud
https://www.statista.com/chart/31901/countries-per-region-with-biggest-increases-in-deepf ake-specific-fraud-cases/ https://www.statista.com/chart/32108/experiences-views-of-experts-on-advanced-identity-f raud-methods/
https://sumsub.com/newsroom/sumsub-research-global-deepfake-incidents-surge-tenfold-fr om-2022-to-2023/
Almars, Abdulqader. (2021). Deepfakes Detection Techniques Using Deep Learning: A Survey. Journal of Computer and Communications. 09. 20-35. 10.4236/jcc.2021.95003.
Rana, Md & Sung, Andrew. (2020). DeepfakeStack: A Deep Ensemble-based Learning Technique for Deepfake Detection. 70-75. 10.1109/CSCloud-EdgeCom
Comments